Contactless fingerprints¶
id3 Finger SDK provides a contactless finger detection and matching component used to detect one or multiple fingerprints from a picture taken with a simple camera.
It takes as input a high resolution picture of 1, 2, 3 or 4 fingers (defined in the FingerPosition Enumeration), extract the image of each finger and compute the corresponding template.
A global matching score can then be computed between a contact-based and a contactless templates, or between two contactless templates, using the compareTemplateRecords() method of the FingerMatcher module.
Attention
The input image must follow some requirements for the system to operate properly :
The fingers must come from the bottom of the image
The image must be flipped, so that the order of the fingers is identical to a contact-based sensor
The flash must be used during the image capture process, and the focus correctly set, to ensure that the minutiae are clearly visible
The image must be at (approx.) 500 dpi. We recommand a Full HD resolution (1080p) to have enough details
The fingers indicated by the FingerPosition enumeration must be visible on the image (no more, no less)
Note
The background does not have to be uniform but it might help the camera to focus on the fingers.
Fingers can be closed or slightly spread apart but should not overlap.
In case of injury on one or multiple fingers, you must select the FingerPosition enumeration accordingly and not show the finger(s) in the image.
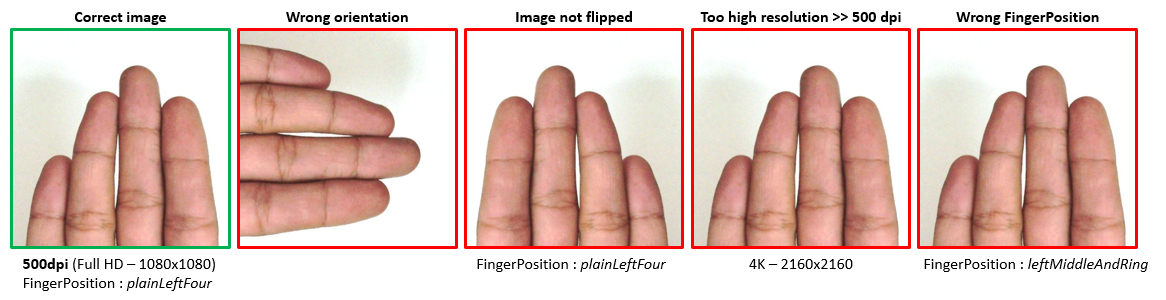
Example¶
Extracting a contactless template and matching against a reference
The example below demonstrates how to generate a FingerTemplateRecord from a contactless image and match it against a reference template.
# Imports
from id3finger import *
# Check if licence is valid
FingerLicense.check_license("./id3FingerSDK_v4.lic")
# Load models
FingerLibrary.load_model("finger_contactless_detector_v1a.id3nn", FingerModel.FINGER_CONTACTLESS_DETECTOR_1A, ProcessingUnit.CPU)
FingerLibrary.load_model("finger_minutia_detector_v3b.id3nn", FingerModel.FINGER_MINUTIA_DETECTOR_3B, ProcessingUnit.CPU)
FingerLibrary.load_model("finger_minutia_encoder_v1a.id3nn", FingerModel.FINGER_MINUTIA_ENCODER_1A, ProcessingUnit.CPU)
# Initialize models
finger_detector = FingerDetector()
finger_extractor = FingerExtractor(thread_count=1)
finger_matcher = FingerMatcher(multiscale_match=True) # The multiscale parameter must be set to True when matching contactless templates
# Load reference template
reference_template_record = FingerTemplateRecord.from_file("./reference_template.bin")
# Load image
image = FingerImage.from_file("./img.jpg", PixelFormat.BGR_24_BITS)
# Flip the image horizontally to ensure that the template is in the right position to be compared to a contact-based acquired template
# This is necessary only if the two templates to match come from different sources (contact-based/contactless)
flip_horizontally = True
flip_vertically = False
image.flip(flip_horizontally, flip_vertically)
# Set the finger position (Here we expect the index, middle, ring and little fingers from a left hand)
image.position = FingerPosition.PLAIN_LEFT_FOUR
image.set_resolution(500)
# Detect fingers
detected_fingers = finger_detector.detect_contactless(image)
# Count the number of detected fingers (should match the number of expected fingers)
num_fingers = detected_fingers.get_count()
print("Number of detected fingers :", num_fingers)
# Extract template of all fingers
contactless_image_record = finger_detector.extract_fingers(image, detected_fingers)
# Create a template record from the contactless template
contactless_template_record = finger_extractor.create_template_record(contactless_image_record)
# Compute the global score between the captured template and the reference
trust_filled_position = False
global_score = finger_matcher.compare_template_records(reference_template_record, contactless_template_record, trust_filled_position)
print("Global score :", global_score)